Turning Chaos into Predictive Power
- November 1, 2024
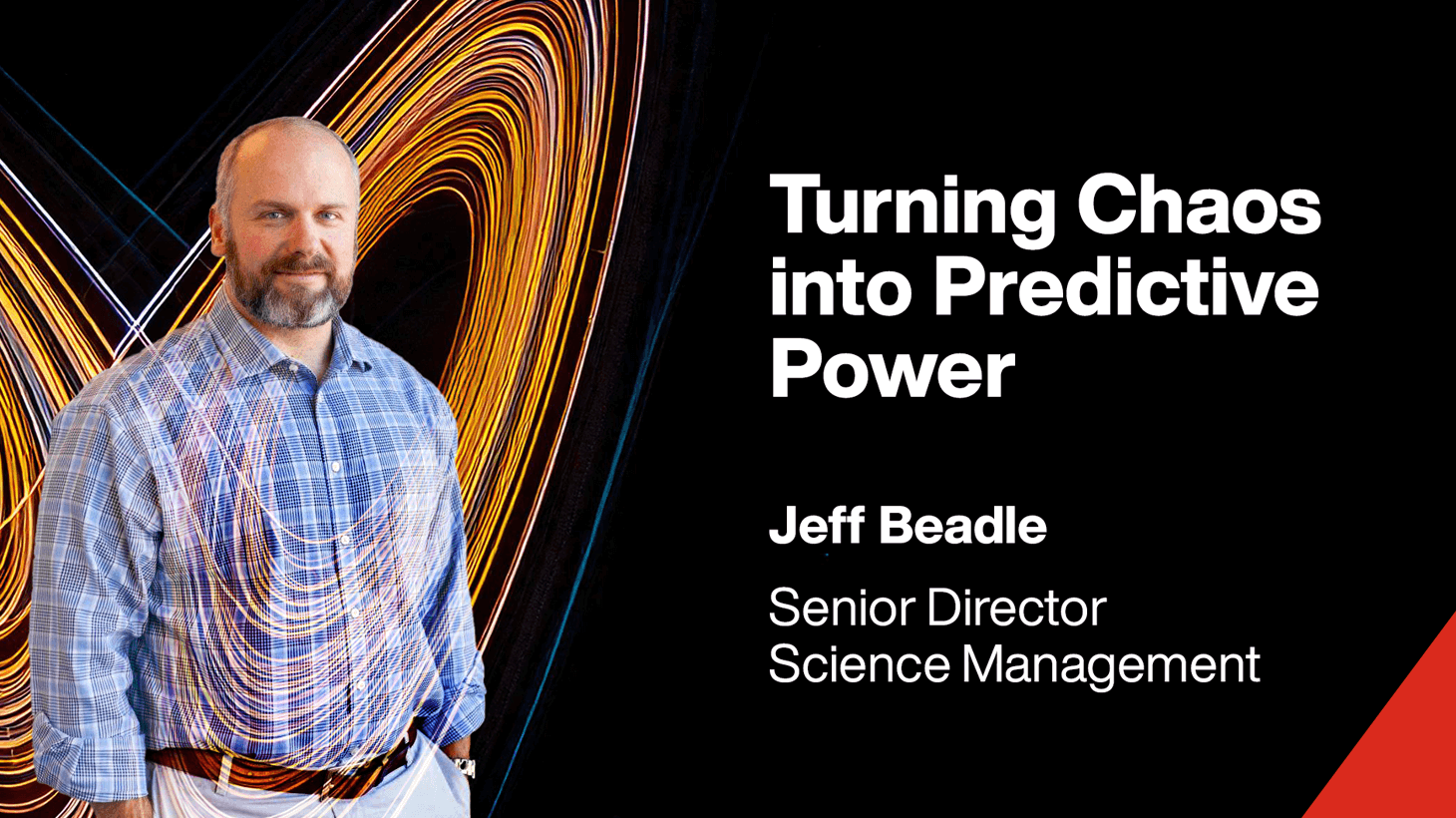
My journey to supply chains began at Georgia Tech in the Applied Chaos Lab, where a sign on the entry door read, “Applied Chaos—Oxymoron or Reality?” It perfectly captured the complexity of what we were working on—chaotic systems. These systems seemed random, but with the right approach, we could uncover the underlying order. One particularly illuminating project involved running electricity through a vibrating ferromagnetic ribbon. At first, the data looked like pure noise, but when we mapped it in 3D, a stable pattern emerged. That’s when I learned that chaos often hides a deeper structure—if you know how to find it.
Today, I see the same principles at work in supply chains. My background in chaos theory taught me not to react to the noise, but to uncover the patterns that drive it. In supply chains, it’s all about turning that unpredictability into something you can control.
When I joined Manhattan 26 years ago, demand forecasting relied on selecting from six different models, each suited to specific situations. This allowed for flexibility, but also required analysts to choose the best model for each case, which sometimes let errors accumulate before adjustments could be made. I saw a different way. By combining the strengths of all six models, our team developed the Unified Forecasting Method (UFM)—a single, continuously adaptive system that captured trends, seasonality, and unexpected spikes in real time.
With the rise of machine learning, UFM evolved into UFM.ai. Now, we don’t just rely on internal data—we integrate external factors like weather forecasts, CDC reports, and even social media to predict demand more accurately. A favorite example of mine is the “Kardashian Effect.” For a beauty retailer, a single tweet from a Kardashian can trigger a massive demand spike. UFM.ai can detect this in real time and ensure stores are stocked before the rush hits.
The power of UFM.ai goes beyond beauty trends. We can apply this predictive power to everything from flu shots to automotive parts, using external data to dynamically adjust forecasts and optimize inventory. It’s no longer about managing chaos—it’s about transforming it into predictive power that will help our clients stay ahead of the curve.
My journey has taught me that chaos isn’t just noise— it’s filled with patterns waiting to be uncovered. By turning chaos into predictive power, we’re helping supply chains anticipate the future, not just react to it.
Learn More About UFM.ai
Manhattan’s enhanced Unified Forecasting Method with ML (UFM.ai) can help keep pace with complex and changing demand patterns.